Ertürk Poyraz | Actionable Insight Derived From Data
Hotel Reservation
Just a couple of months ago, as I was returning to my hometown from Istanbul, I had a problem in the bus: I had bought my ticket from my hometown to Istanbul. Luckily they were able to fix it on the go. The host of the bus said "it's lucky you bought the ticket from our website otherwise we wouldn't be able to cancel it."
​
I thought it must be really difficult for companies like this to manage their reservations, tickets etc.
So... I prepared a mock analysis project to see if I can help "Hotel AsMarti" with managing their reservations:
​
​
Table of Contents
-
Find appropriate data, Kaggle
-
Prepare and clean the data, Excel
-
Analyze and create result tables, BigQuery (sql)
-
Visualize the results, Tableau
-
Discussion
Find Appropriate Data
We use Kaggle to search for Datasets.
After filtering the results for good usability score; we find a hotel reservation dataset with cancelation info.
The dataset has Attribution 4.0 International (CC BY 4.0) license and 10.0 usability score.
Our dataset consists of 19 features (columns) and 36276 observations (rows).
​
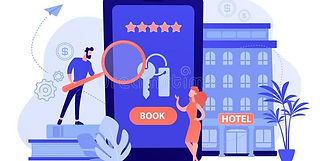
Booking_ID, no_of_adults, no_of_children, no_of_weekend_nights, no_of_week_nights, type_of_meal_plan, required_car_parking_space, room_type_reserved, lead_time, arrival_year, arrival_month, arrival_date, market_segment_type, repeated_guest, no_of_previous_cancellations, no_of_previous_bookings_not_canceled, avg_price_per_room, no_of_special_requests, booking_status,
Prepare and Clean
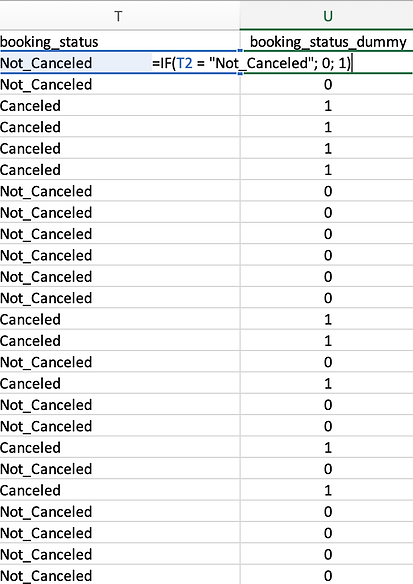
Our dataset consists of 19 features (columns) and 36276 observations (rows).
After checking for
-
Irrelevant data
-
Structural errors
-
Possible outliers
-
missing data
​Using filters, cell formatting and various excel formulas...
We begin our transformations:
-
Create Dummy variables for booking_status using IF function
-
Create a check-in date feature with the exact date as YYYY-MM-DD, which is suitable for BigQuery. Using CONCAT function
​​
Then the file is saved in "csv" format for the following parts.​
Analyze and Create Result Tables
We upload our analysis-ready data to BigQuery and start our queries in order to gain insight into what can be done to manage canceled reservations and reservations in general.

#num of cancelled booking, total booking and the percentage
SELECT
COUNTIF (booking_status = 'Canceled') AS num_of_cancelations,
COUNT (booking_status) AS total_bookings,
(COUNTIF (booking_status = 'Canceled')) / (COUNT (booking_status)) AS percentage
FROM `portfolio1-376720.project_1.reservations`
#num of cancelled booking, total booking and the percentage grouped by year
SELECT
DISTINCT arrival_year,
COUNTIF (booking_status = 'Canceled') AS num_of_cancelations,
COUNT (booking_status) AS total_bookings,
(COUNTIF (booking_status = 'Canceled')) / (COUNT (booking_status)) AS percentage,
FROM `portfolio1-376720.project_1.reservations`
GROUP BY arrival_year

#num of cancelled booking, total booking and the percentage grouped by month
SELECT
DISTINCT arrival_month,
COUNTIF (booking_status = 'Canceled') AS num_of_cancelations,
COUNT (booking_status) AS total_bookings,
(COUNTIF (booking_status = 'Canceled')) / (COUNT (booking_status)) AS percentage,
FROM `portfolio1-376720.project_1.reservations`
GROUP BY arrival_month
ORDER BY arrival_month
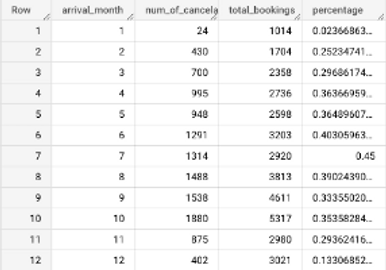
#num of cancelled booking, total booking and the percentage grouped by segment type
SELECT
DISTINCT market_segment_type,
COUNTIF (booking_status = 'Canceled') AS num_of_cancelations,
COUNT (booking_status) AS total_bookings,
(COUNTIF (booking_status = 'Canceled')) / (COUNT (booking_status)) AS percentage,
FROM `portfolio1-376720.project_1.reservations`
GROUP BY market_segment_type
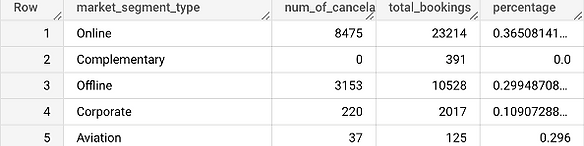
SELECT
CORR(lead_time, booking_status_dummy) AS corr_lt_bs FROM `portfolio1-376720.project_1.reservations_table`
#Correlation of guest being repeated and cancelation
SELECT
CORR(repeated_guest, booking_status_dummy) AS correlation_repeat_cancelation
FROM `portfolio1-376720.project_1.reservations_table`


Following some additional queries, we begin to visualize our findings with Tableau
Visualize Insights
Tableau is able to connect to numerous data sources, by giving permission, we connect BigQuery to Tableau and use the tables above and their relations as inputs for the viz.

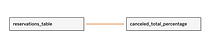
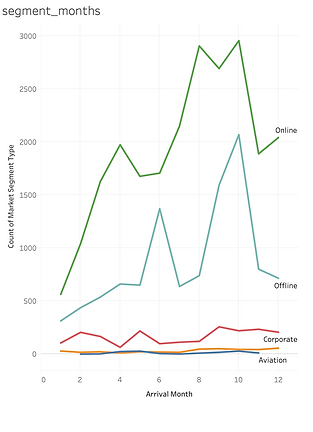
Number of visitors from each segment type, month to month

Number of cancelation for each segment type, and the percentages
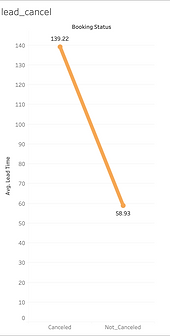
Average lead time for the canceled and not_canceled reservations

Change of cancelation ratio according to price (steady)
(green = not_canceled)
(red = canceled)
(size_of_square = avg_price)

Cancelations over the years

Discussion
Hotel Asmarti has 32% total cancelation rate. Which is a real bummer.
To manage reservations in a way that maximizes profit, a feasible strategy looks like this:
​
-
Initiate overbooking for reservations with 160+ days of lead time. Canceled reservations has an average of 140 days of lead time, while not canceled reservations has an average of 59. Almost all the reservations leading 160+ days can be expected to cancel. An exception route can be presented to corporates.
-
Deploy cancelation fee, optimize according to market segment and lead time: Online reservations lead the way in cancelations with 36%. Targeting them, reservation can be sold for higher prices with the choice of cancelation. Further optimization require website traffic data. Such data would enable the AsMarti hotel to create marketing and consumer personas and get the most out of cancelation fee.
-
Corporates almost never cancel their reservations, they bring approximately the same amount of money with offline and online segments but they constitute a minor proportion for AsMarti. Marketing campaigns targeting corporate groups and incentivized payment plans for these groups will increase their proportions which is preferable for the hotel
-
Expensiveness or the luxury of the reservation (number of special requests, car parking space, luxury room etc.) is irrelevant from chance of cancelation. It would be preferable for AsMarti for them to correlate negatively: Start "push notifications", e-mail marketing and phone calls to make sure "big" reservations are not cancelled.
-
As the number of total bookings increase, the percentage of cancelation increased to; this might indicate a lack of attention on the hotel's part. Further investigate the communication channels of the hotel to make sure customer experience is spotless.